News
better business decisions
Posted 1 year ago | 6 minute read
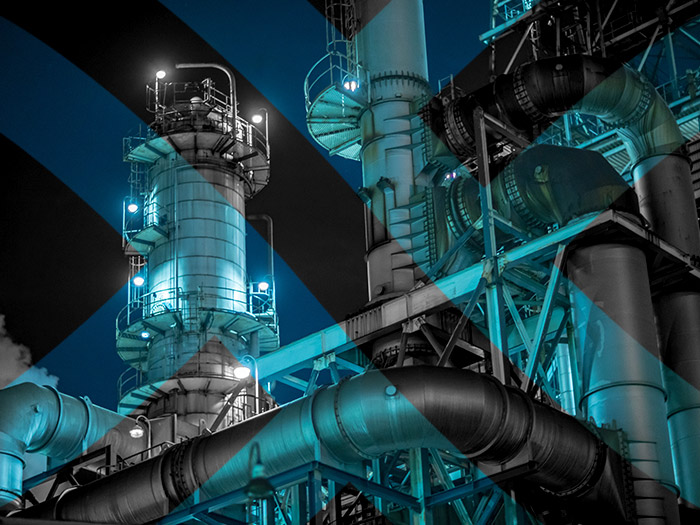
Energy-oriented production planning with time-dependent energy prices
In today’s energy-conscious world, businesses are increasingly looking for ways to reduce their energy consumption and costs. One approach is to adopt energy-oriented production planning, which involves considering energy consumption and prices when making production decisions.
When it comes to energy, value is determined by three key factors: price and quantity and time. When and how you use energy is just as important as the price you pay for it and how much you use. Ultimately, the ability to be flexible about when you use energy represents both a value and a cost. This means that some types of industry are able to schedule production to align with periods of lower energy prices and to optimise their production processes to reduce emissions and even gain revenues.
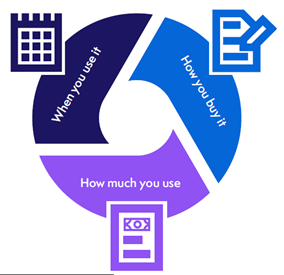
The importance of time-dependent energy prices
Manufacturing processes typically consume significant amounts of energy, impacting both operational costs and environmental footprint. Traditional production planning approaches have often assumed that energy prices are constant. This means many production planning methodologies prioritise factors like output maximisation or lead time minimisation, without considering energy consumption. Integrating energy use into production schedules is crucial for achieving sustainable and cost-effective operations.
In many markets, energy prices vary significantly over time, depending on factors such as supply and demand, time of day, and day of the week. Failing to account for these time-dependent prices can lead to production decisions that result in higher energy costs. The emergence of dynamic energy pricing models further intensifies the need for energy-aware production planning. These models introduce significant price fluctuations based on grid conditions, creating opportunities to shift production activities to coincide with lower energy cost periods. But efficiently adapting production schedules to these dynamic prices while satisfying other operational constraints can present a complex optimisation problem.
Challenges
There are a number of challenges associated with planning production around energy prices, including:
- Data requirements: Accurate hourly or even minute-by-minute energy price data is essential for effective planning. Additionally, detailed information about the energy consumption profiles of individual machines and processes is required.
- Computational complexity: The multi-dimensional optimisation problem involving scheduling, energy price variations, and operational constraints can be computationally challenging, especially for large production systems.
- Trade-offs and conflicting objectives: Balancing energy cost minimisation with other objectives like production deadlines, machine capacity limitations, and inventory management can lead to complex trade-offs and require carefully defined optimisation goals.
Benefits: Cement plant optimisation
Harnessing the power of big data and site-specific information from an extensive network of sensors and controllers, GridBeyond’s optimisation model successfully reduced energy consumption in industrial production without affecting quality or production targets, saving our client (a cement manufacturing facility in Great Britain) £3,694,827 per year in energy costs while ensuring its production schedules were met.
Challenge: The site was using power to operate throughout the day, despite the cost of power fluctuating across settlement periods. Energy flexibility was found in the silo assets without impacting overall product quality or operations – providing an opportunity to make cost savings on electricity and generate revenue for the business.
Solution: By reducing production when at or near maximum capacity, the three silos on site and using Robotic Trading, energy flexibility was traded in the highest revenue markets. This meant the site earned revenue from flexibility offered in grid programmes in addition to avoiding the peak price power periods and reducing overall production costs. Production from the kiln and further downstream in the operational process was not impacted.
Results: By forecasting market prices, shifting load, and trading, GridBeyond’s solution saved £3,694,827 annually, accompanied by a substantial reduction of Scope two emissions per year.
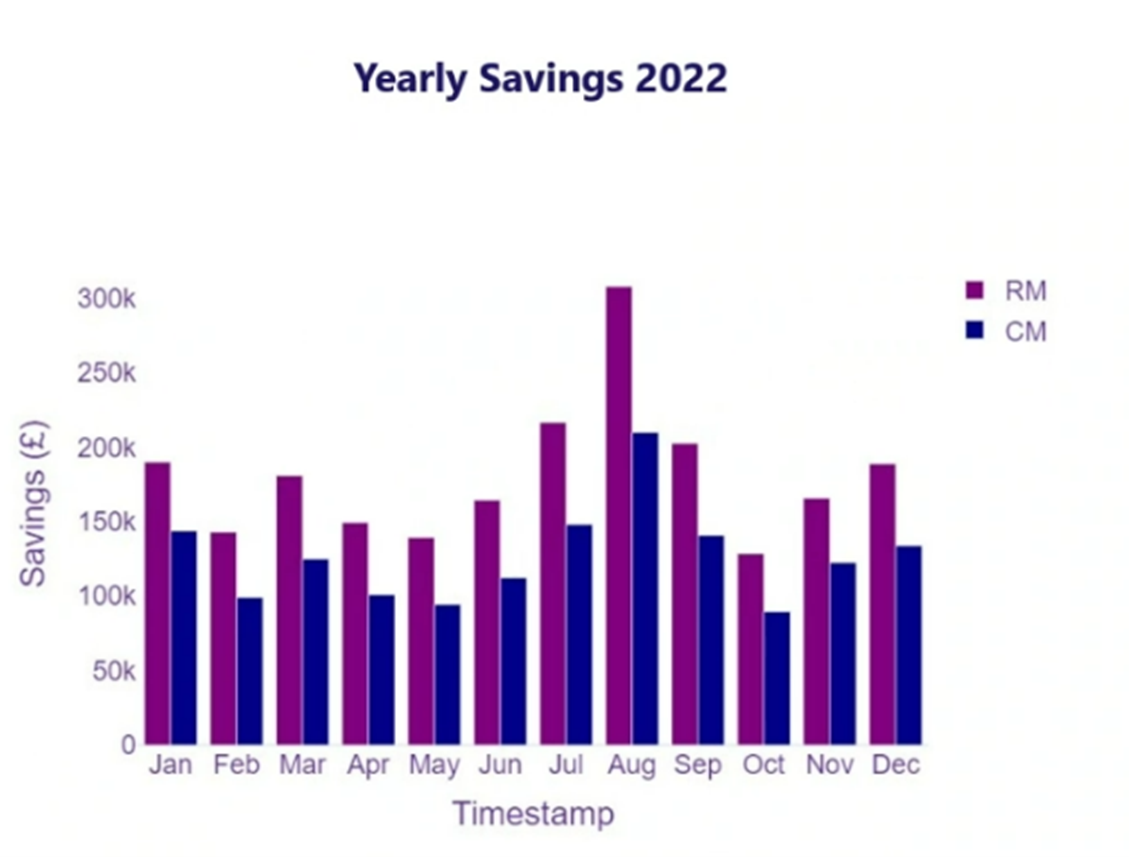
The model incorporates the following key elements:
- Decision variables: Binary variables are used to represent whether each job is processed in each time period. Integer variables indicate the quantity of each product produced in each period.
- Objective function: Minimises the total energy cost, calculated by multiplying the energy consumption of each activity by the corresponding time-dependent energy price and production quantity.
- Constraints: Operational constraints, such as job precedence relationships, machine capacities, and production deadlines, are incorporated.
- Energy consumption: The model utilises energy consumption profiles specific to each activity and machine.
The model provides a systematic approach to find the most cost-efficient production schedule under time-dependent energy prices. It allows for incorporating various operational constraints and optimisation priorities and the model can be adapted to accommodate complex production systems with diverse equipment and product types.
Advanced capabilities for maximum savings
High energy bills have a direct impact on production costs, and at GridBeyond, we offer a comprehensive suite of capabilities tailored to address this challenge. Our advanced features include:
- Digital Twin: of your plant and production schedule to forecast revenues in participation in the energy markets
- Scheduling optimisation: Calculating the best economic production schedule, factoring in constraints including daily production targets, energy costs, raw and cement mill load, silo storage capacity and kiln load
- Forecasting: Precise forecasting of energy demand and market prices with up to 98% accuracy
- Stacking Up: Consolidation of diverse energy resources including solar PV, battery storage and industrial load for efficient microgrid utilisation
- Monitoring: Real-time insights into energy consumption and emissions from operations for baselining against carbon targets and predictive maintenance
- Flexibility monetisation: Leveraging grid flexibility for additional revenue streams
- Market integration: Seamless integration with all available grid programmes and energy markets for maximum revenue and savings
- Asset finance – CapEx & OpEx free battery financed by our funding partner and/or on-site solar – financed through a PPA to transform your site into a sustainable micro-grid
Production scheduling with time-dependent energy prices is an important tool for businesses that want to reduce their energy consumption and costs. While there are challenges associated with this approach, there are also a number of solutions available.
At GridBeyond our AI-powered forecasting, combined with automated control solutions and a smart energy management platform, presents a promising approach to optimise production schedules and minimise energy costs, providing a robust framework for addressing the increasing pressures of energy efficiency and price dynamics.
Harnessing the power of big data and site-specific information from an extensive network of sensors and controllers, GridBeyond’s optimisation model is designed to deliver the best economic production schedule for your facility. Our platform employs AI and machine learning, ensuring that hard constraints such as asset capacity and daily production targets are met while optimising against energy costs, asset load, and any on-site generation or storage technologies.