News
better business decisions
Posted 4 years ago | 6 minute read
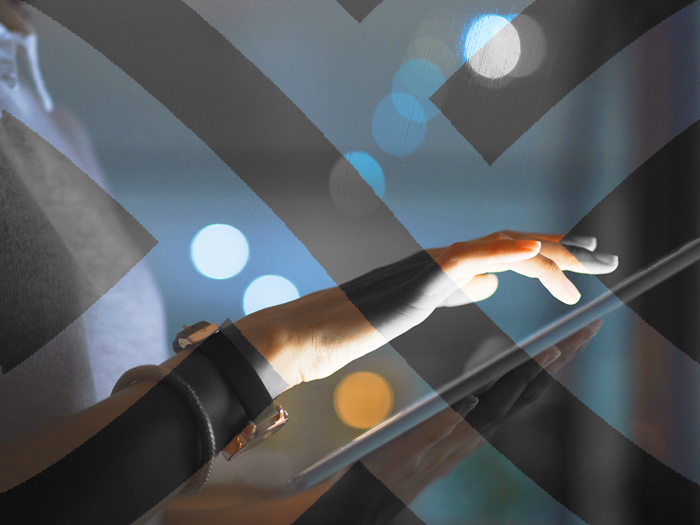
Machine Learning – all hype or golden opportunity?
The machine learning era is here, and energy is often cited as one of the most relevant sectors for the application of this technology, bringing numerous benefits for all players in the value chain. But why is it important, and is now the time to start embracing this game-changing technology?
Matching electricity consumption with the right level of generation is crucial, as excess power cannot be stored, unless converted to other forms, for example by using storage technologies. The difficulty lies in the non-linearity and volatility of real-time energy supply and demand, which is highly susceptible to changes in external factors. Both underestimating and overestimating energy demand could affect the balance on the grid and possibly cause blackouts.
Clearly, there are tangible benefits in closely monitoring the system. With the advent of machine learning, predicting future energy consumption becomes not only increasingly possible but more accurate. There’s no doubt that machine learning presents a huge opportunity for the energy sector. But what is machine learning?
What is machine learning?
In essence, machine learning is a type of artificial intelligence (AI) where software has the ability to learn without being explicitly programmed. The software is made using algorithms and statistical models. For them to work, they require good data, which they process, and learn from. It is a process similar to how humans learn but much faster and more scalable.
When forecasting energy demand and supply, the following types of variables are important to consider:
- Calendar data: season, hour, bank holidays, etc.
- Weather data: temperature, humidity, rainfall, etc.
- Company data: the price of electricity, demand. historical consumption of electricity, asset health and demand
- Social data: economic and political factors that a country is experimenting with.
Based on big data and existing knowledge, machine learning technology determines the most likely outcome from every modelled scenario, while tuning after each event to further improve future decision-making. Its ability to understand the correlation between individual events and their impact on electricity supply and demand, means guesswork is replaced by a structured, evidence-based, repeatable approach.
A holistic approach
For a large energy user, the benefits of an intelligent energy management strategy powered by AI technologies include:
- Price Optimisation and Trading: An intelligent energy management system helps businesses to decide how to arrange site schedules to take optimum advantage of low price bands for the energy they buy. I&C can use onsite generation or storage assets to ensure constant access to the energy supply, minimise the risk of blackouts, and to sell back energy to the grid at a profit. A global leader in paper and packaging used two of its onsite CHP generators to sell unused power to a power supplier. The company was soon earning around £10k in average additional monthly revenue without any capital expenditure or impact on operational processes.
- Action Behind the Meter: Where a large energy user has the capacity to change their demand, generate or store energy, intelligent energy management enables energy end-users with flexibility on their sites, whether it is embedded in their operational assets, comes from onsite generation or storage, participation in a suite of grid balancing services. This represents a profit opportunity as businesses are being financially incentivised by the grid for their support One catering supplier in the UK generates £300,000 of revenue annually from demand side response as part of their intelligent energy management strategy.
- Advanced Asset Management: Predictive maintenance, benchmarking, and performance analysis of the onside assets can all be built into businesses’ intelligent energy management system, ensuring cost reduction and more operational efficiency. Extensive research by GridBeyond shows that a fault detected early results in approximately £8k in maintenance costs and production loss, whereas reactive maintenance can cost around £48k. That’s a 500% reduction in cost.
But the key to the development of a successful holistic strategy lies in having accurate data inputs and experienced software developers and engineers to programme the algorithms.
In a recent interview GridBeyond’s Head of Trading and Optimisation, Paulo Sobral, said:
“Many businesses haven’t considered the use of AI technologies, such as machine learning, as part of their energy strategy given the high cost outlay in data and the expertise needed to analyse and build your algorithms. But if done well, the revenues can be significant. It’s important to note that as markets are increasingly moving closer to real-time, energy users need to jump on opportunities as fast as possible. So, the end of the chain is a trading robot that conducts the transaction in the most optimal circumstances and at the best price.”
When combined, machine learning and robotic trading can bring real benefits. But what’s the risk?
Risk & reward
As with anything that relies on a forecast, there is some potential for risk.
“Usually, If we take more risks, we can get more benefits. The key to using machine learning effectively is ensuring that you have accurate data inputs. At GridBeyond, the way we do this is by combining over 60 external data inputs with onsite information and analysis. This allows us to build algorithms that assign the risk that the client is comfortable with, and secondly, to monitor that risk”, Sobral said.
There’s no denying that energy consumers could participate in energy trading without technology in place. However, this would require some serious internal communications systems and dedicated expert resources to fulfil the market participation requirements from moment to moment. And even with this structure in place, it would be unlikely for a business to reap returns to its full potential. So, technology is the key.
At GridBeyond, we use machine-learning to collate all the relevant data from the sites and the markets. The Point platform then automates participation in the most lucrative markets, meaning the clients don’t have to do a thing. We also take a holistic approach to energy markets and operations, so whilst trading is one element, the platform also uses this sophisticated technology to enter client’s profile into demand response services and dynamic asset optimisation programmes.
If you have any questions about the potential for your site, contact our team, or to learn more about the complimenting services offered by GridBeyond’s intelligent energy platform, download the energy services brochure.