News
better business decisions
Posted 2 months ago | 5 minute read
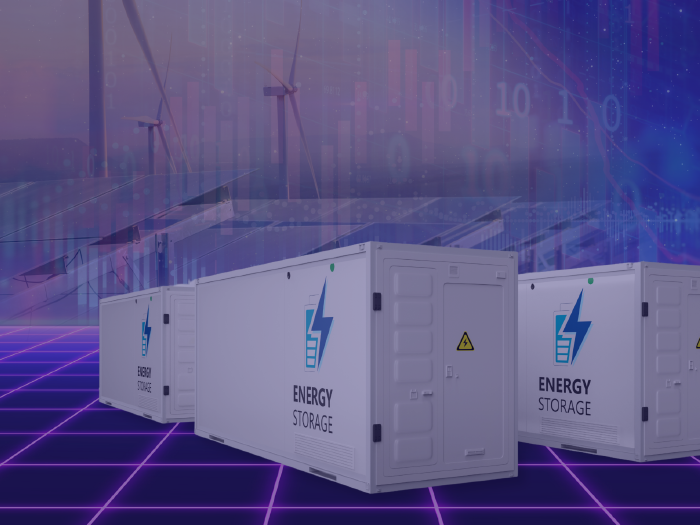
Optimizing battery science for maximum efficiency and profitability
Battery storage is revolutionizing the energy sector, playing a pivotal role in grid stability, renewable energy integration, and cost efficiency. But understanding the science behind battery optimization, degradation, and forecasting is essential for maximizing its potential.
A fundamental principle in battery economics is balancing revenue generation with battery wear and tear. Every charge and discharge cycle contributes to degradation, impacting long-term profitability. The goal is to maximize financial returns while minimizing unnecessary battery strain for long term revenues. This article explores why marginal cost matters, the key factors at play and how GridBeyond’s approach to battery management maximizes lifetime returns for battery assets.
What is marginal cost?
Marginal cost is the incremental cost of producing or consuming one more unit of a good or service. In the energy sector this means that the marginal cost of a power plant is the cost of generating an additional megawatt hour of electricity. It depends largely on the primary energy source, but also on the type of power plant and the situation in which the electricity is to be generated. This means a key aspect of battery optimization is relating the potential revenue it can earn against the associated marginal cost of its degradation.
Several factors influence this including:
- Depth of charge and discharge: adjusting how much a battery is charged or depleted can extend or shorten its lifespan
- State of health (SoH) indicates the overall condition of a battery relative to its original capacity. Monitoring SoH ensures reliable participation in energy markets by maintaining performance efficiency
- State of charge (SoC): SoC refers to how much energy is stored at any given time. This metric is crucial for determining when a battery should charge or discharge to align with high-revenue opportunities
- Market pricing: Aligning charging and discharging with energy price fluctuations
Battery degradation is a natural process influenced by usage patterns, temperature, and charge cycles. By factoring in variables like depth of discharge and state of charge, the system can fine-tune its approach to marginal cost. For example, it might adjust how deeply the battery is discharged or charged in a given cycle to minimize wear and tear, extend the battery’s lifespan, and ultimately lower the cost per cycle. By combining advanced battery science modelling to simulate wear and tear and real-time data from Energy Management Systems (EMS), asset operators and owners are able to track actual performance, predict and mitigate degradation, ensuring batteries remain efficient and cost-effective over time . This granular level of control helps ensure that revenue or savings generated by the battery—whether through arbitrage, grid services, or energy cost reductions—consistently surpasses the marginal cost, delivering a strong return on investment.
The role of forecasting
Energy price forecasting is a critical aspect of energy storage optimization since it can be used to adjust battery operations in real-time. Traditional forecasting models rely on historical trends, but advanced methods leverage:
- Probabilistic forecasting, which a range of potential outcomes, unlike traditional forecasting methods that rely on single-point estimates. This improves risk management, as decisions are made based on likelihoods rather than assumptions and market responsiveness, by adapting to shifting energy dynamics in real-time
- Machine learning algorithms to analyze multiple market variables and to continuously refine predictive models based on real-world data. This also allows for different forecasting models to compete against each other to determine the most accurate approach, allowing for future adaptation to market changes, ensuring forecasts remain relevant
- Advanced monitoring techniques utilize real-time machine learning models to detect disruptions as they happen, power flow analysis to identify transmission bottlenecks, and predictive algorithms to anticipate potential failures. that can impact energy storage operations.
Combined, machine learning models detect intricate patterns, anticipate price spikes and dips with high confidence. Instead of providing a static price forecast, probabilistic predictions are generated that account for market volatility, offering a strategic advantage in energy trading.
At GridBeyond, our team blends machine learning with deep regulatory expertise, ensuring our forecasts align with evolving energy policies.
Whether it’s identifying subtle shifts in capacity or pinpointing stress points from overuse, the interplay between advanced modeling and EMS data ensures we’re not just reacting to degradation after it happens, but actively managing it over time. This technique empowers users to extend battery life, optimize performance, and maximize value, regardless of the battery’s make or model. In addition, we leverage AI and machine learning to analyze power flows, voltage fluctuations, and equipment performance, detecting disruptions instantly. Unlike traditional monitoring methods that rely on manual oversight, our technology adapts its forecasting parameters dynamically, ensuring our predictions remain precise even in volatile grid conditions.
Our advanced battery optimization and forecasting solutions set a new standard in energy storage profitability. By mastering marginal cost optimization, battery health monitoring, and cutting-edge price forecasting, we empower businesses to maximize returns while ensuring long-term sustainability. With our AI-driven approach, we don’t just predict the future—we help you profit from it.
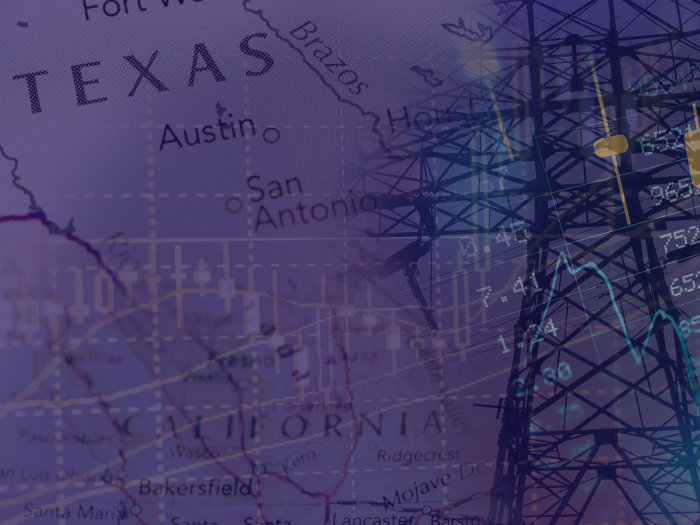
ERCOT and CAISO market trends in 2024 and successful bid optimization
In this paper we examine market trends in ERCOT and CAISO impacting power storage and what makes a successful bid optimization strategy.
Learn more